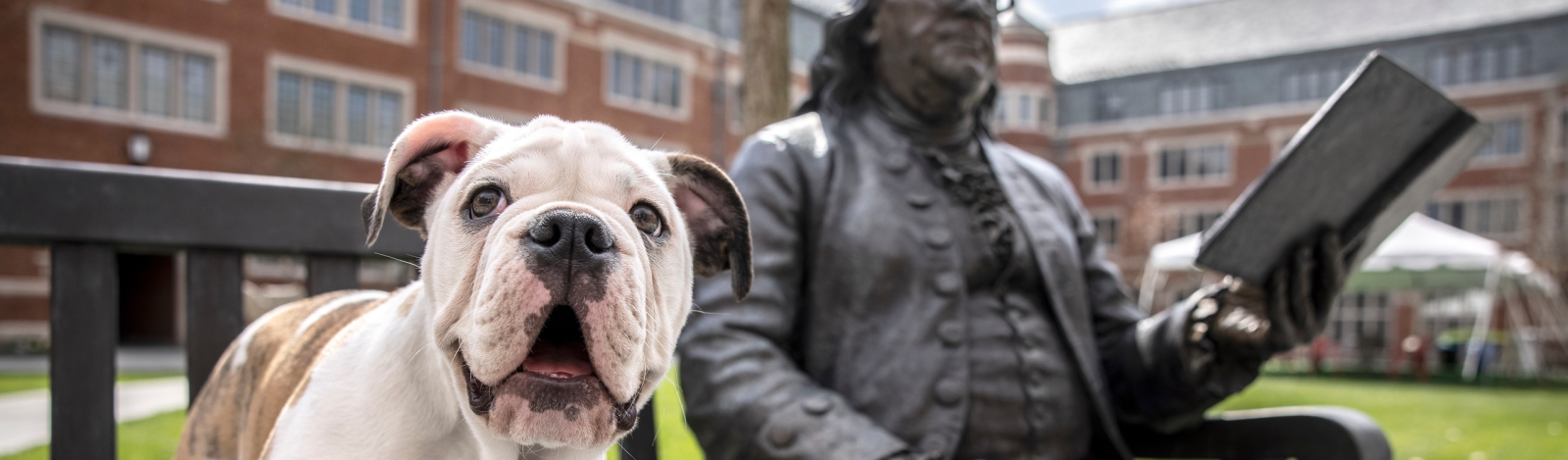
Description
Health informatics (HI) is an in demand field comprising applied research and the practice of informatics across clinical and public health domains. Informatics researchers develop, introduce, and evaluate new biomedically motivated methods in areas as diverse as data mining, natural language or text processing, cognitive science, human-computer interaction, decision support, databases and algorithms for analyzing large amounts of data generated in public health, clinical research and genomics/proteomics.
In this online program, you will develop the skills and knowledge around data, health and informatics needed to attract attention from recruiters and hiring managers in the fast growing healthcare industry.
You will gain a thorough understanding of the field of health informatics and its various subfields, including research, laboratory/precision medicine, imaging, and artificial intelligence. You will also explore the themes that serve as the foundation for different areas of biomedical informatics, including clinical and neuro-informatics.
Throughout four courses and hands-on projects, you will leverage the expertise of faculty from the Division of Health Informatics at the Yale School of Public Health, learning from real-world projects and live sessions with groups of high-caliber peers.
By committing 6-8 hours of online study per week for about 7 months, you can earn a Yale-issued Health Informatics MasterTrack Certificate that can help you in your future graduate studies or professional pursuits in both public health departments (e.g. CDC, local government) and the private sector (e.g. organizations that employ computer scientists and mathematicians/statisticians). After successfully completing the MasterTrack, you will also be eligible for conditional credit if accepted into the Yale School of Public Health’s Master of Public Health (MPH) graduate degree program.
Upon successful completion of this MasterTrack Certificate, you will be eligible to earn 2 conditional credits counting towards the Yale School of Public Health’s MPH program if accepted.
Program Takeaways
- Take the next step in your biomedical, clinical, and public health career by exploring innovative approaches to data and knowledge management in a live, simulated environment taught by Health Informatics faculty at the Yale School of Public Health.
- Complete industry-relevant hands-on projects to build your portfolio
Courses
Health Informatics is a diverse and varied field. This course provides an introduction to informatics topics pertinent to healthcare and serves as a foundation for other informatics-related courses. Through lectures, discussions, and real-world applications, students will gain foundational knowledge in clinical information systems, biomedical data, health data standards, electronic health records, clinical decision support, health data security and telemedicine.
Upon completion of this course, students will be able to:
- Describe the design, function, and evaluation of clinical information systems
- Examine the role and utility of data standards in Health Informatics
- Discuss privacy and confidentiality issues around clinical data
- Analyze important frameworks for clinical decision making and how this knowledge integrates into clinical decision support systems
This course picks up where Course 1 leaves off and introduces additional key informatics topics pertinent to healthcare. Through lectures, discussions, and real-world applications, students will survey a variety of informatics subfields including artificial intelligence, bioinformatics and precision medicine, data warehouses and registries, research informatics, electronic quality metrics and IT evaluation, software tools, and open science.
Upon completion of this course, students will be able to:
- Discuss the challenges and opportunities of artificial intelligence in healthcare
- Explain the factors that influence the delivery of personalized medical experiences
- Analyze the function and role of data warehouses and registries
- Examine the impact of clinical research informatics
- Explore and analyze innovations in digital health
This course provides an introduction to common unifying themes that serve as the foundation for different areas of biomedical informatics, including clinical and neuro-informatics. Through lectures, tutorials, discussions, and applied practice, students will learn how to build databases and utilize computational tools for use in biomedical research.
Upon completion of this course, students will be able to:
- Explore Python and Google Colab
- Define the SQL and NoSQL database models
- Discuss the challenges of data integration
- Identify the pros and cons of data warehouses and federated databases
- Examine semantic web as a NoSQL database technology
- Analyze ontology-driven applications
The course is designed for students with coding knowledge and an advanced level of experience using biomedical software.
This course builds off of Course 3 by taking a deeper dive into topics in biomedical informatics and data science. Through lectures, tutorials, discussions, and applied practice, students will explore biomedical natural language processing, data mining, machine learning, modeling of biological systems, and high performance computation in biomedicine.
Upon completion of this course, students will be able to:
- Define natural language processing (NLP) and clinical NLP
- Process and parse unstructured text narratives and transform the text into a structured format using natural language processing methods
- Build and evaluate different data classification models
- Enhance performance of the data models using different techniques such as feature selection and ensemble learning
- Apply basic python programming skills to better understand natural language processing and machine learning
- The course is designed for students with coding knowledge and an advanced level of experience using biomedical software